Precision in Medical Segmentation with Compound Loss Functions
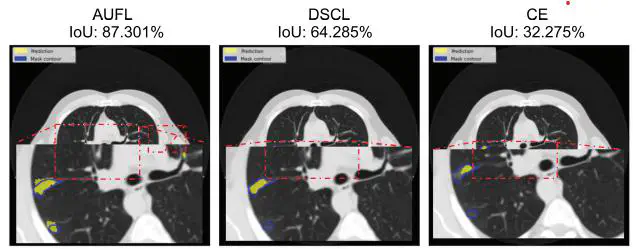
We’re excited to share our latest publication! Our new study explores how different loss functions affect the precision of medical image segmentation using deep learning. Specifically, we evaluate three types—AUFL, DSCL, and CE—applied to the detection of COVID-19-related lung lesions in CT scans, using advanced architectures like TransUNet, ViT, and U-Net.
The results are striking: the compound loss function AUFL stands out for its accuracy and stability, consistently outperforming the others—especially in segmenting small lesions like ground-glass opacities (GGOs), which are common in this condition.
This work marks a milestone in our research line focused on improving deep learning models for clinical-grade segmentation, with real-world applications in AI-assisted diagnosis and treatment. Our goal: to ensure reliable and reproducible segmentations, even in lower-resolution modalities like CBCT.
Advancing precision in medical image segmentation: A performance analysis of loss functions for COVID-19 lung infection segmentation in computed tomography images is one of the outcomes of musicgenia, a project funded by grant CPP2021-008491 from MICIU/AEI/10.13039/50100011033 and the European Union through NextGenerationEU/PRTR.
Curious about why AUFL performs best, and how these loss functions compare across architectures and modalities? Reach out and we’ll be happy to explain.